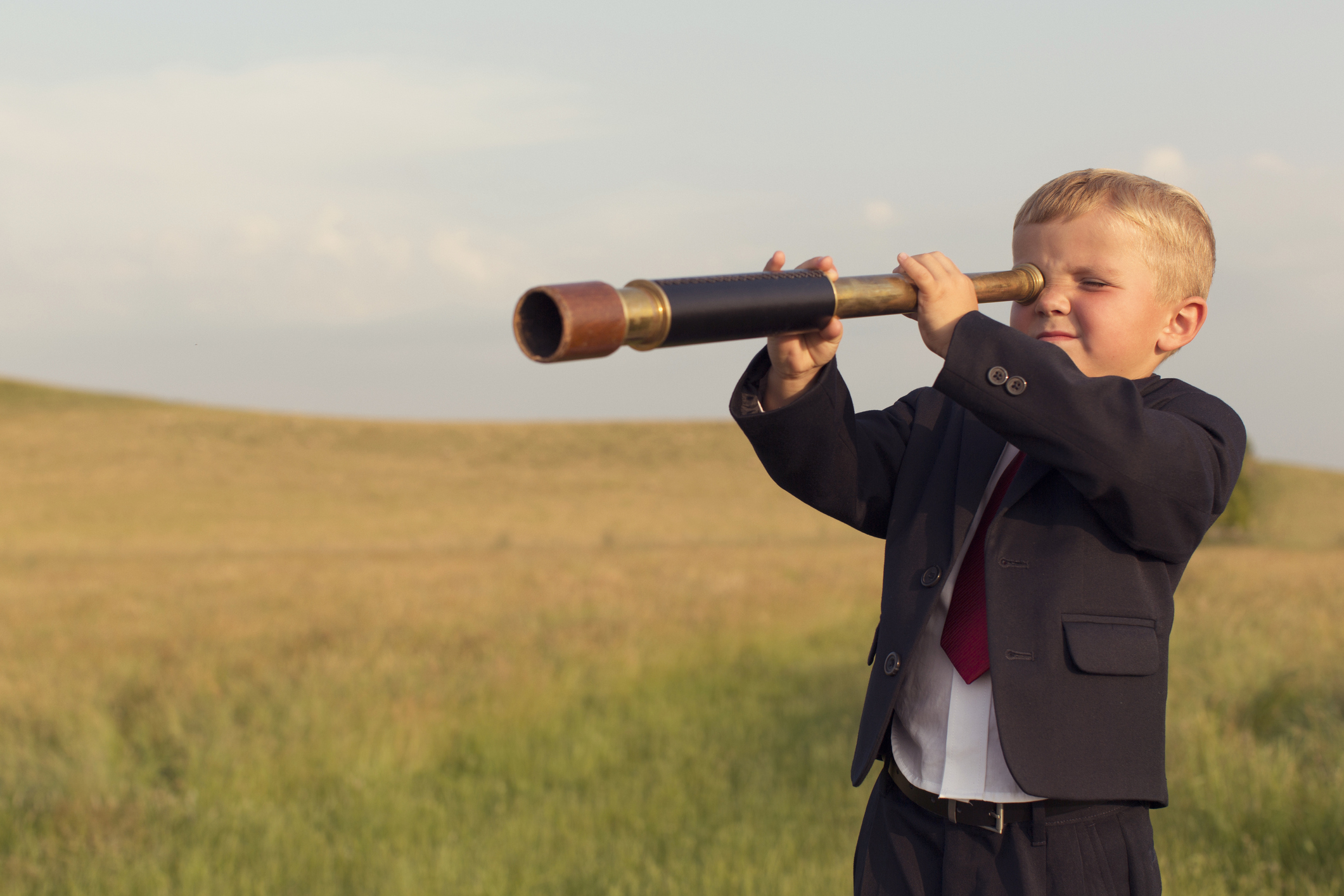
Neural Machine Translation
Machine Translation (MT) technology has taken another leap forward. This time, it’s a big leap forward. Instead of relying on the phrase-based statistical approach, neural MT simulates the interconnected network of the brain to generate translations. Several companies have unveiled neural MT technology, including Google, Microsoft, and Facebook. Try it out here. For many language pairs, neural MT generates output that is markedly higher quality than statistical MT. Google’s neural MT even seems to create its own “interlingua,” between language pairs never seen before, that is, an abstract representation of language within the network itself.
Neural MT is not a silver bullet, at least not yet. Currently, neural MT generally outperforms statistical MT in language pairs such as Chinese, Korean, Japanese, Turkish, and Arabic. However, statistical MT still seems to outperform for Romance languages such as Spanish, Portuguese, and French. For that reason, independent MT developers aren’t completely abandoning the statistical approach. Neural MT is being implemented as part of an overall architecture of neural, statistical, and in some cases, rules-based machine translation engines. The architecture then employs predictive analytics to decide which engine will yield the best result for any given piece of content.
In some ways, MT won’t surpass the quality of human translation anytime soon. In other ways, it already has. MT engines excel in consistent use of correct terminology, whereas a human translator is prone to human error. For this reason, human translators are working increasingly side-by-side with MT to cover their weak points while at the same time improving their productivity. Whether by using MT output in its raw form or by using “post-edited” MT output, MT is enabling translation buyers to translate more content than ever for a compelling price.
Quality Benchmarking
“What is quality?” The localization industry may have a more concrete answer to this question with the development of quality benchmarks. These benchmarks will begin to materialize for certain content profiles based on real data gathered from translation buyers and sellers. The industry-supported technology driving this trend is the TAUS DQF (Dynamic Quality Framework). The vision behind DQF is to standardize the methods and tools for quality evaluation, aggregate the scores and measurements and make these available as industry-shared metrics.
DQF can be implemented on any project by enabling a plugin available in most translation tools and translation management systems. DQF measures time the translator spends on each segment. At project completion, a reviewer marks errors based on a set of criteria specified for the content profile, (for example, web marketing or help documentation). The scores are then sent anonymously to the DQF central database, where all scores and productivity rates are stored for each content profile.
As DQF is enabled for more projects, industry-wide quality benchmarks for certain content profiles will begin to materialize. Buyers and sellers of translation will then be able to implement these benchmarks into their SLAs, simplifying quality expectations for everyone.
New Business Models
Paying by the word has and continues to be an industry standard pricing model. However, paying by the word may not be the best model for services like transcreation and multimedia. For transcreation, consider Netflix, where transcreating 5 words of a TV show title could take days of research and wordcraft. For multimedia, consider all the production hours required by motion graphics artists, audio engineers, voiceover artists, and post-production QA. The cost of a project like this doesn’t depend much on word count.
However, as long as the video meets certain requirements in length and complexity, it’s possible to create a package pricing model, where buyers buy a pack of localized videos for a static cost per video. This model is results-driven, and it simplifies budgeting for both the buyer and seller. Expect this model and others like it to gain traction in the industry in 2017.
Related Guides – free!
Introduction to Machine Translation (MT)
Localization – “Cut Costs, Not Corners”
Transcreation Guide: Fast-Tracking your Transcreation Project
Client Review Checklist